- NanoBits
- Posts
- AI for Everyone š: F for Foundation Models
AI for Everyone š: F for Foundation Models
Nanobits AI Alphabet

EDITORāS NOTE
Hello Fellow AI Adventurers,
Since November 2022, all of us have been bustling the Grand AI Expo, surrounded by mind-boggling demos of talking chatbots, image generators, and even AI-composed music and videos. Itās like a machine that never stops innovating! But have you ever wondered what's powering all this creativity?
If AI is a Lego masterpiece, then foundation models are the building blocks that make it all possible.
These massive AI models, trained on gargantuan amounts of data, are like the Swiss Army knives of artificial intelligence. They can write poems, generate images, translate languages, and even predict protein structures.
But what exactly are foundation models? How do they work their magic? And what are the implications of having such powerful tools at our fingertips?
In this edition of the AI Alphabet, we're pulling back the curtain on "F" ā for Foundation Models. We'll uncover:
The story behind the name "Foundation Model" and why it's on everyone's lips.
How these models are trained and the secret behind their versatility.
The ways foundation models are transforming AI development, making it faster and more accessible.
The good, the bad, and the potentially ugly side of this powerful technology.
DEFINE: FOUNDATION MODELS
Why are Foundation Models Hard to Define?
Yesterday when I was researching the concept of foundation model and how it came to be, I almost got lost in the vast array of AI lexicons. Foundation models are often misunderstood due to the lack of a universal definition for "AI" itself and the evolving nature of terminology.
However, a shared understanding of these powerful tools is crucial for effective communication and decision-making across the public, policymakers, industry, and media.
Recognizing the unique features of FMs is essential for responsible development and targeted regulation since their versatility suggests that the underlying issues might be magnified across various applications.

Image Credits: Ada Lovelace Institute
What is a Foundation Model?
FMs are a type of AI technology that are trained on vast amounts of data that can be adapted to a wide range of tasks and operations, such as text, image or audio generation.
They can be standalone systems or can be used as a ābaseā for many other applications. For example, the LLM called GPT works as the foundation model of ChatGPT.

Image Credits: Nvidia
TYPES OF FOUNDATION MODEL
Established Terms
Foundation Model, also known as GPAI (General Purpose AI), can overlap with other popular concepts like āgenerative AIā and ālarge language models (LLMs).
Generative AI
Itās a broad term used to describe AI systems whose primary function is to generate content, in contrast with other AI systems designed for other tasks, such as classification and prediction.
It is important to note that not all generative AI are foundation models. Generative AI can be narrowly designed for a specific purpose. Some generative AI applications have been built on top of foundation models, such as OpenAIās DALLĀ·E or Midjourney, which use natural language text prompts to generate images.

Image Credits: TechSpot
Note that generative AI tools are not new, nor are they always built on top of foundation models. For example, generative adversarial networks (GANs) that power many Instagram photo filters and deepfake technologies have been in use since 2014.

Image Credits: Brain Glitch on Medium
Large Language Models (LLMs)
LLMs are AI systems used to model and process human language. They are called ālargeā because they have hundreds of millions or even billions of parameters, which are pre-trained using a massive amount of text data.
LLMs can predict the next most likely word in a sentence given the previous paragraph. This is commonly used in applications such as Google Docs or Gmail, which make suggestions as you are writing.

Image Credits: Reddit User
Since GPT-4, LLMs have increasingly become multimodal ā that is, they can use multiple inputs and generate multiple outputs.
Googleās PaLM-E, an embodied multimodal language model, is capable of visual tasks (such as describing images, detecting objects, or classifying scenes), and robotics tasks (such as moving a robot through space and manipulating objects with a robotic arm).

Image Credits: LinkedIn
More Contested Terms
Frontier model refers to cutting-edge AI models with capabilities surpassing current advanced models. These models are often characterized by their superior performance and potential to tackle a broader range of tasks.
However, the definition of "frontier model" is still evolving, and there's no universally agreed-upon way to measure which models qualify. Currently, the computational resources required for training are sometimes used as a proxy, but this may change as technology advances.
Artificial General Intelligence, often referred to as "strong AI," represents a hypothetical future state where AI systems possess human-level cognitive abilities across various domains.
While companies like OpenAI and Google DeepMind aspire to create AGI, it remains a theoretical concept with no current examples. The definition of AGI is also contested, with some describing it as machines capable of performing any task a human can, while others focus on broader capabilities like reasoning and learning from experience.
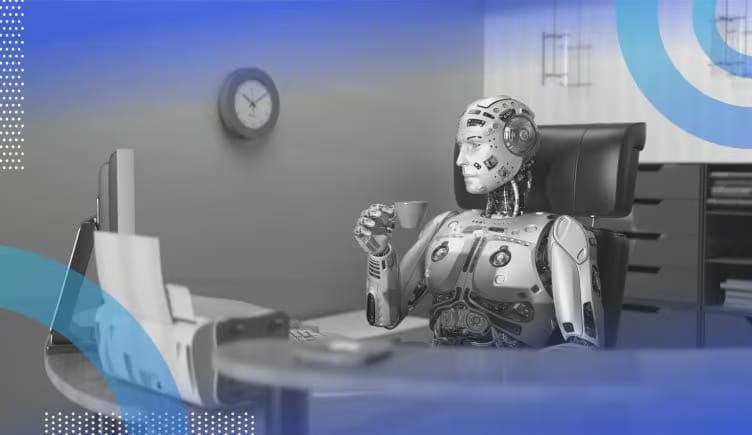
Image Credits: Built In
Summary of Relationships between Terminologies:
These terms are interconnected, with AI being the foundational concept, and the other terms representing specific technologies and applications that build upon each other.
Artificial Intelligence: The overarching field encompassing all other terms.
Foundation Models: Large, versatile models that serve as a base for various AI applications, including LLMs and generative AI.
LLMs: A subset of AI focused on natural language understanding and generation.
Generative AI: A subfield of AI that includes techniques and models for creating new content.
Stable Diffusion: A specific method within generative AI for generating images from text, utilizing principles from foundation models and LLMs.
HOW DO FOUNDATION MODELS WORK?
So, what's happening behind the scenes of these AI powerhouses? Foundation models operate on a fascinating blend of scale and adaptability.

In Image: Selection of FM Developments since Sept. 2023;
Image Credits: AI Foundation Models Technical Update Report by CMA
Here's how they work:
Pre-Training: Foundation models start by learning the general patterns and structures of language, images, or other data through a process called pre-training. This involves feeding them massive amounts of raw data, like the entire internet, to identify statistical relationships and connections.

In Image: Image-Caption Pre-training; Image Credits: Catalyzex
Fine-Tuning: After pre-training, foundation models are fine-tuned for specific tasks using a smaller, more targeted dataset. This allows them to adapt their general knowledge to specific applications, like generating creative writing or summarizing medical documents.
Modality: Foundation models can be unimodal, handling only one type of data (like text), or multimodal, processing multiple types of data (like text and images). This flexibility allows them to tackle a wide range of tasks and applications, from language translation to image recognition.

Image Credits: AI Foundation Models ā Initial Report by CMA
APPLICATIONS OF FOUNDATION MODEL
FMs have a wide range of potential applications across different industries, including healthcare, finance, customer service, and creative industries. They can be used for tasks like language translation, content creation, medical diagnosis, and more, offering the potential to drive significant technological advancements and efficiency improvements.

Image Credits: Ada Lovelace Institute
LLMs MADE IN INDIA
Krutrim
Krutim AI by OLA is a generative AI assistant that converses in 10+ Indian languages, aiming to revolutionize how Indians interact with technology, breaking down the linguistic and cultural barriers that often hinder AI adoption.

Image Credits: CEO Vines
OpenHathi
The first publicly available Hindi Large Language Model by Sarvam AI trained on Hindi, English, and Hinglish data.

Image Credits: Sarvam AI
Bhashini
Bhashini is a government initiative to bridge the digital divide by developing AI and language technology services for various Indian languages.

Image Credits: CivilsDaily
Navarasa 2.0
Navarasa 2.0 by Telugu LLM Labs is an advanced iteration of the Gemma series language models, that supports an extensive suite of 15 Indian languages along with English.

Image Credits: Hugging Face
Project Indus
An initiative by Tech Mahindra to develop a pure Hindi Large Language Model, incorporating 37 dialects and aiming to expand to other languages.

Image Credits: Web3Cafe
Other LLMs
Odia Llama: Fine-tuned for the Odia language, enhancing its digital presence and respecting Odisha's cultural heritage
Kannada Llama: A powerful model designed for the Kannada-speaking community, improving AI's understanding of the language
Tamil Llama: A language model specifically designed for Tamil, enhancing its capabilities in handling Tamil text
BharatGPT: A GenAI platform for Indians, supporting 14+ languages and ensuring data sovereignty
Dhenu Llama 3: An Indian Language AI model for farmers
THE FUTURE OF FOUNDATION MODELS
To date, there has been significant investment in organizations that develop FMs from a range of businesses, including venture capital.

Image Credits: AI Foundation Models ā Initial Report by CMA [Dated 18th Sept 2023]
The future of foundation models is closed-source.
Centralization vs. Decentralization:
The future of foundation models is a battleground between centralization and decentralization. While open-source models like Llama-3 have recently gained traction, their long-term viability faces challenges. The narrative of AI decentralization, championed by open-source advocates, clashes with the reality of scaling laws favoring powerful, closed-source players.

Image Credits: NLP Cloud
The Economics of Open-Source AI:
Open-source AI, while seemingly free, incurs significant costs for developers in terms of inference, GPU management, and hosting. These costs, coupled with the lack of a direct feedback loop between production usage and model training, make open-source models less attractive for model builders seeking financial returns. As Meta, a major proponent of open-source AI, prioritizes its own interests, the tipping point for discontinuing open-sourcing might arrive sooner than expected.
The National Security Implications:
Open-source AI raises concerns regarding national security. Releasing model weights to the public could empower adversaries and competitors, enabling them to develop their own advanced AI capabilities. This potential misuse of AI for malicious purposes poses a significant risk, particularly in areas like cyberattacks, disinformation campaigns, and critical infrastructure manipulation.
The future of foundation models is likely to be shaped by the delicate balance between open access and safeguarding sensitive technology.
THE GOOD, BAD, AND UGLY
Despite their broad potential, foundation models pose many challenges, including the following:
Bias. Because foundation models stem from only a core few technologies, inherent biases due to social or moral issues in those few models might spread through every AI application.
System. Computer systems are a key bottleneck for scaling model size and data quantity. Training foundation models might require a prohibitively large amount of memory. The training is expensive and computationally intensive.
Data availability. Foundation models need access to large amounts of training data to function. If that data is cut off or restricted, they don't have the fuel to function.
Security. Foundation models represent a single point of failure, which makes them a viable target for cyber attackers.
Environment. It takes a large environmental toll to train and run large foundation models, like GPT-4.
Emergence. The outcomes of foundation models can be difficult to trace back to a particular step in the creation process.
LAST THOUGHTS
We've explored the inner workings of foundation models, these massive AI systems that will reshape our world. Their potential for good is immense, but so are the challenges and uncertainties they pose. As we navigate this uncharted territory, here are a few questions to ponder:
Who holds the power? Will foundation models further centralize AI development in the hands of a few powerful players, or will open-source initiatives democratize access and foster innovation?
What about the unintended consequences? As foundation models become more integrated into our lives, how will we mitigate the risks of bias, misinformation, and even potential misuse for malicious purposes?
Will foundation models unlock the path to AGI? Are we witnessing the early stages of a technological revolution that could lead to artificial general intelligence, or is that just a far-off dream?
The future of foundation models is a story that's still being written. It's a tale of potential, but also of responsibility. As we witness the progress of this new emerging technology, it's up to us to ensure that these powerful tools are used for good, not harm.
So, dear readers, what do you think?
Are foundation models a force for good, a Pandora's box of unintended consequences, or something in between?
Share your thoughts and join the conversation in the comments.
Until next time, keep exploring the exciting world of AI!
Thatās all folks! š«”
See you next Saturday with the letter G

Image Credits: Cartoon Stock
If you liked our newsletter, share this link with your friends and request them to subscribe too.
Check out our website to get the latest updates in AI
Reply